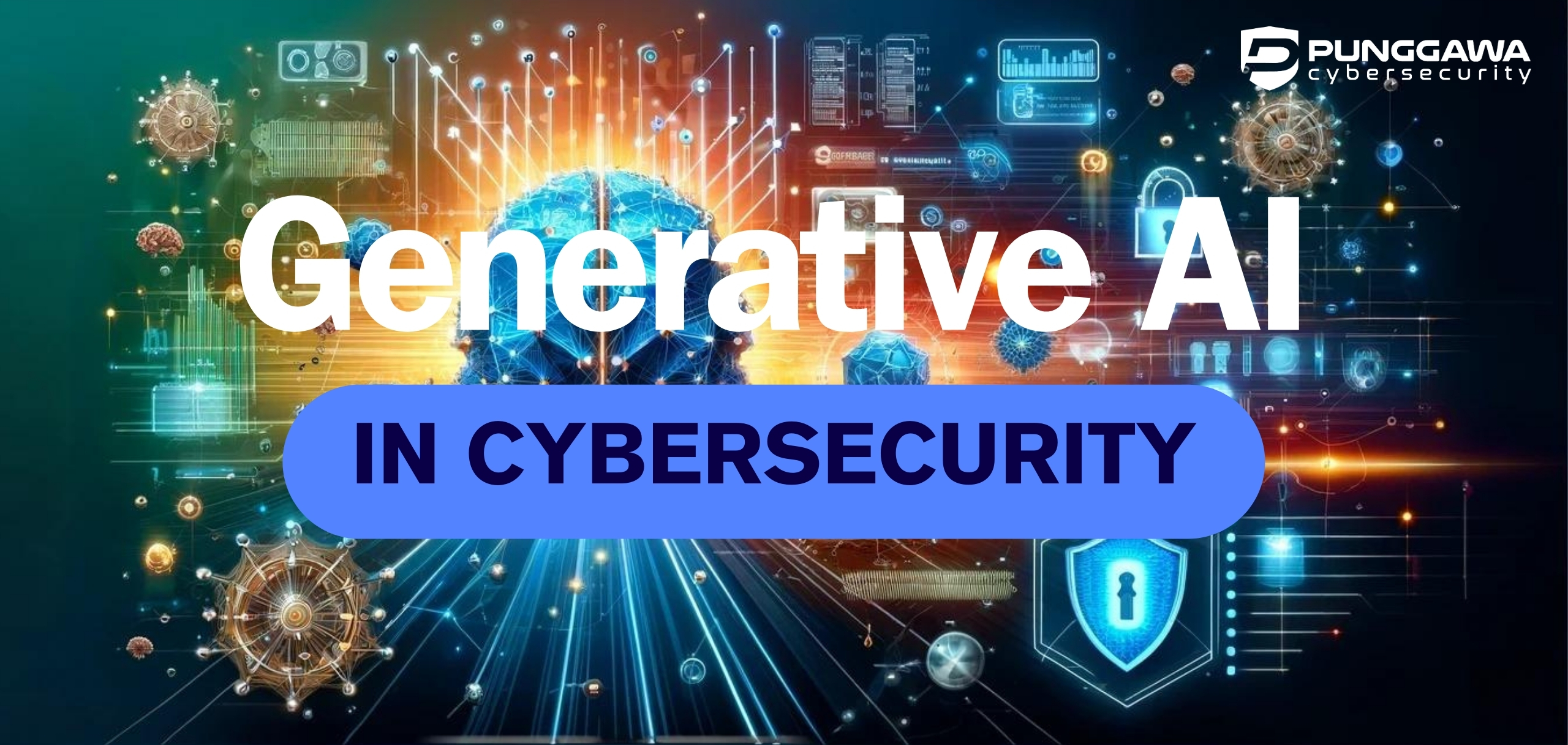
Generative AI in Cybersecurity
In the ever-changing cybersecurity landscape, Generative AI emerges as a game-changing technology with the potential to revolutionize threat detection, system integrity assessments, and defense mechanisms. At its heart, Generative AI employs algorithms that learn from extensive datasets and generate new content or patterns that reflect the learned data. This ability, fueled by advancements in machine learning and neural networks, intense learning models like GPT (Generative Pre-trained Transformer), and variational autoencoders, paves the way for creating realistic simulations and scenarios for comprehensive cybersecurity training and testing.
The application of Generative AI in cybersecurity is becoming crucial as cyber threats grow more sophisticated. Traditional security measures often need help keeping pace with the rapid development of new attack vectors and strategies. Generative AI bridges this gap by enabling the automated generation of potential threat scenarios, allowing cybersecurity systems to anticipate and prepare for a broader range of possible attacks. This proactive stance is vital in a landscape where data breaches’ cost and frequency continue escalating, pushing organizations to adopt more dynamic and adaptive security practices.
Moreover, integrating Generative AI into cybersecurity tools can significantly enhance the efficiency of security operations centers (SOCs). By automating the creation of threat intelligence, such technologies can provide SOCs with enhanced situational awareness and faster response capabilities. The ability of Generative AI to analyze historical data and predict future incidents offers a strategic advantage, ensuring that security measures are not just reactive but also predictive. This shift from a defensive to a preemptive security posture is instrumental in building resilient systems that can withstand the advanced cyber threats of the digital age.
In the ever-changing cybersecurity landscape, Generative AI emerges as a game-changing technology with the potential to revolutionize threat detection, system integrity assessments, and defense mechanisms. This ability, fueled by advancements in machine learning and neural networks, paves the way for creating realistic simulations and scenarios for comprehensive cybersecurity training and testing.
Impact of Generative AI on Cybersecurity
Reshaping Threat Detection and Response
Generative AI is revolutionizing the threat detection and response field by introducing systems that are not only faster but also more adaptable to the evolving landscape of cyber threats. Traditionally, threat detection systems relied heavily on signature-based methods that required known patterns of malicious activity to identify threats. However, Generative AI enables behavior-based detection systems, which learn from data to identify abnormal patterns that could indicate a security breach. This shift significantly reduces the time to detect and mitigate threats, as AI systems can analyze vast quantities of data in real time, spotting anomalies that human analysts might miss.
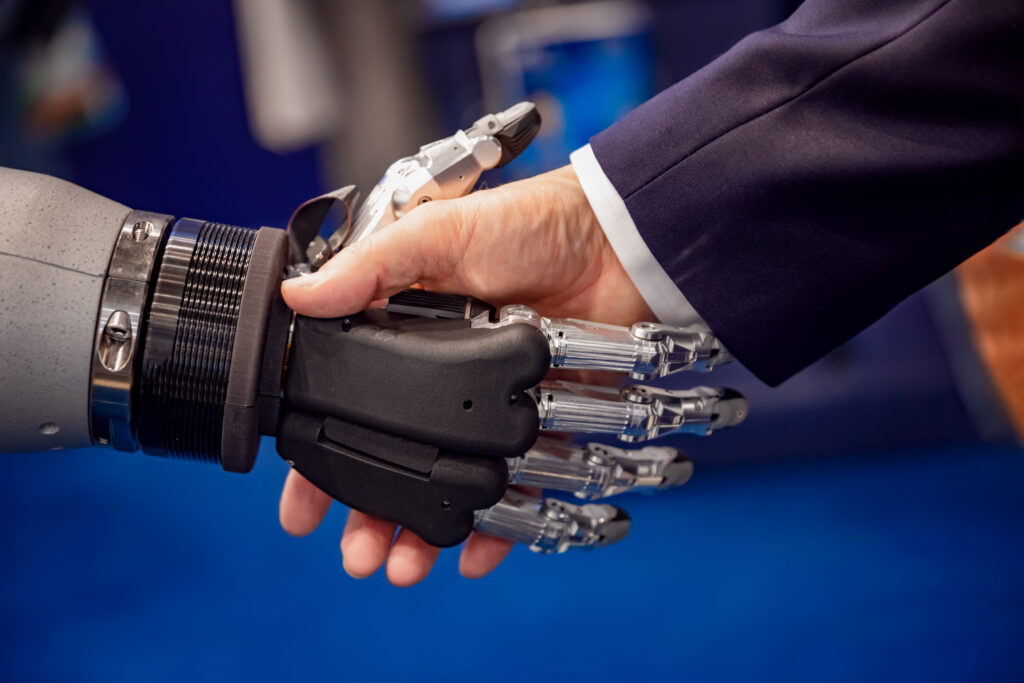
Moreover, Generative AI enhances response strategies by simulating various attack scenarios and predicting their potential impacts on network systems. This allows cybersecurity professionals to prepare more effective mitigation strategies that are proactive rather than reactive. For instance, AI-generated insights can help develop automated response actions for common attack patterns, ensuring that systems can respond instantly to threats, thus minimizing potential damage.
Additionally, these AI technologies facilitate continuous learning and adaptation. Generative AI models are continuously trained to refine their threat detection and response capabilities as new data becomes available. This dynamic approach is crucial in a landscape where attackers constantly evolve their strategies. Generative AI helps maintain a robust defense posture that grows with emerging threats by enabling ongoing adjustments to security protocols and responses.
Dual Roles of Generative AI
Generative AI plays a dual role in cybersecurity by enhancing security measures and introducing new challenges. On the positive side, these technologies can dramatically improve the efficiency and effectiveness of security protocols. For example, Generative AI can be used to create sophisticated cybersecurity training simulations for IT staff, preparing them to handle a variety of attack scenarios. This training can be tailored to an organization’s specific needs, allowing employees to experience realistic attack simulations and learn how to respond effectively.
However, the capabilities of Generative AI also present new challenges. The same technologies used to protect systems can be exploited by attackers to create advanced malware, phishing attacks, and other malicious tools. This raises significant concerns about an arms race in cybersecurity, where defenders and attackers leverage AI to outmaneuver each other. The ease with which Generative AI can generate convincing phishing emails or create malware that can adapt to different systems underscores the need for robust AI security measures and ethical guidelines.
These challenges require a balanced approach to using Generative AI in cybersecurity. Organizations must consider the technological implications and the ethical and security risks associated with deploying these powerful tools. Ensuring that Generative AI is used responsibly and with adequate safeguards is crucial to harnessing its benefits while mitigating its risks.
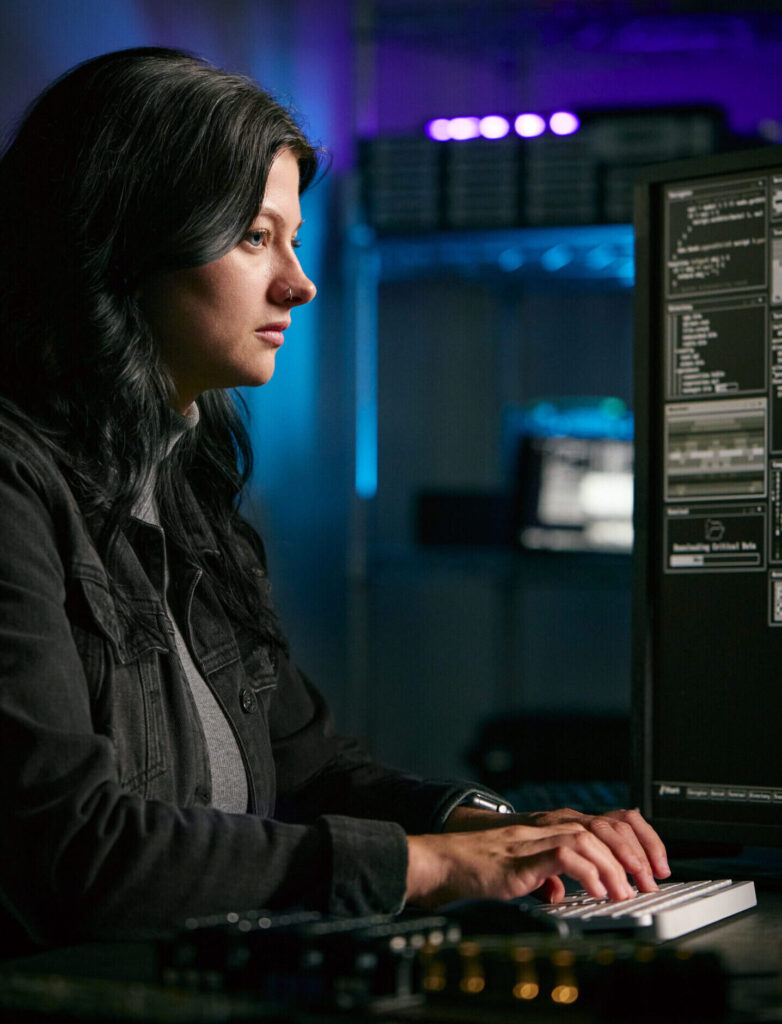
Enhancements in Phishing Detection
The specific advancements in phishing detection using Generative AI are particularly noteworthy. By analyzing patterns from massive datasets of email traffic, AI models can identify subtle cues that indicate phishing attempts, which traditional systems might overlook. These models are trained to detect variations in language, sender behavior, and other metadata that correlate with phishing emails. As a result, Generative AI systems can alert users to potentially malicious emails with greater accuracy and reduce the number of false positives, which are shared with more straightforward filtering techniques.
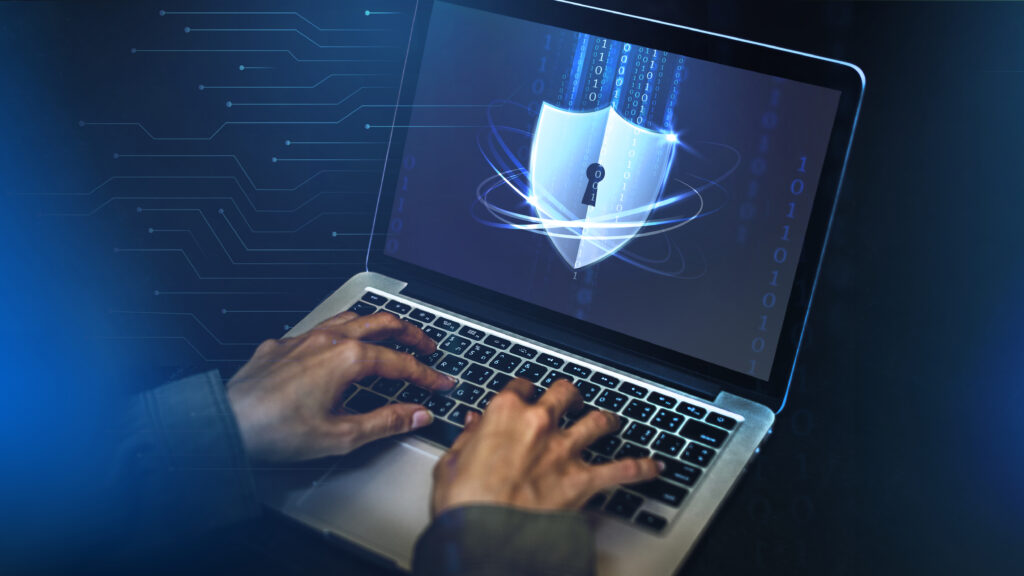
Moreover, Generative AI can test the resilience of an organization’s email systems by generating realistic but harmless phishing attempts. This proactive testing helps identify vulnerabilities in an organization’s email filters and user practices, allowing for timely improvements. It also serves as practical training for employees, increasing their awareness and ability to recognize sophisticated phishing attempts.
Lastly, improving AI capabilities leads to better adaptation against evolving phishing techniques. As cybercriminals develop new methods to bypass security measures, Generative AI models continuously learn from these attempts, improving their predictive accuracy. This creates a dynamic defense system that evolves in response to new threats, ensuring that organizations can maintain high levels of security against phishing attacks.
Leveraging Generative AI for Enhanced Cybersecurity Practices
Cybersecurity analysts can harness the power of Generative AI to revolutionize their daily operations, enhance efficiency, and improve the accuracy of their threat detection and response mechanisms. By integrating Generative AI tools into cybersecurity frameworks, analysts can automate routine tasks, predict potential threats, and focus on strategic decision-making and complex problem-solving.
Automated Threat Detection and Analysis: One of the primary strategies for integrating Generative AI involves automating the detection and analysis of security threats. For example, AI systems can continuously monitor network traffic and analyze patterns to identify anomalies that may indicate a security breach. These systems can be trained on historical data to recognize the signatures of various cyberattacks, from DDoS attacks to sophisticated ransomware campaigns. Cybersecurity analysts can quickly isolate and mitigate threats by automating these processes before they cause significant damage.
Predictive Threat Intelligence: Generative AI can also develop predictive capabilities that forecast future threats based on current trends. This is achieved by analyzing vast data from various sources, including dark web monitoring, hacker forums, and past security incidents. For instance, a Generative AI model might predict an upcoming spike in phishing attacks targeting the financial sector based on recent discussions observed on underground forums. Armed with this predictive intelligence, cybersecurity teams can proactively strengthen their defenses and educate their users, significantly reducing the potential impact of such attacks.
Case Study: Enhancing Incident Response with AI Simulations: Consider a hypothetical scenario where a financial institution faces increasingly sophisticated phishing attacks. By implementing Generative AI, the institution’s cybersecurity team can simulate various phishing scenarios to test their email filters and employee readiness. The AI could generate realistic phishing emails that mimic those identified in recent breaches, allowing the team to measure the effectiveness of their current defenses and train their employees in a controlled, risk-free environment. The insights gained from these simulations can be used to fine-tune policies and improve training modules, making the institution more resilient against attacks.
Streamlining Compliance and Reporting: Finally, Generative AI tools can aid in regulatory compliance and reporting by automating the collection and analysis of compliance data and generating compliance reports. For organizations subject to stringent regulatory requirements, AI can ensure that data handling and security procedures meet industry standards and that any deviations are quickly addressed. This saves time and reduces the risk of human error in compliance processes.
Expanding the Cybersecurity Arsenal: Six Pivotal Use Cases of Generative AI
Incorporating Generative AI into cybersecurity practices is not merely an enhancement of existing protocols but a revolutionary step towards a more secure digital world. Generative AI provides indispensable tools in the modern cybersecurity landscape by simulating, predicting, and automating responses to cyber threats. This section explores six critical use cases where Generative AI makes significant inroads, offering innovative and essential solutions for protecting digital assets in an increasingly complex cyber environment.
Generative AI’s capabilities are varied and vast, from fortifying threat intelligence to refining security training. Each use case demonstrates the technology’s versatility and highlights its potential to transform the field of cybersecurity. By leveraging these AI-driven tools, organizations can achieve more precision in their security measures, reduce human error, and respond more swiftly to potential threats.
1. Threat Intelligence Generation
Generative AI significantly enhances cybersecurity systems’ capability to generate actionable threat intelligence. By simulating various attack scenarios, these AI models help predict potential attack strategies and tactics that adversaries might employ. This proactive approach allows organizations to prepare and strengthen their defenses against possible future threats. Moreover, continual learning from new and evolving threats enables these systems to stay updated, ensuring that the threat intelligence they generate is relevant and timely, thus fortifying the organization’s defenses against ever-evolving cyber threats.
The effectiveness of Generative AI in threat intelligence is underscored by its ability to synthesize information from diverse sources into coherent threat narratives. This not only aids in anticipating attacks but also in understanding potential attackers’ tactics, techniques, and procedures (TTPs). Such comprehensive intelligence is crucial for developing strategic responses and updating security protocols to prevent breaches before they occur.
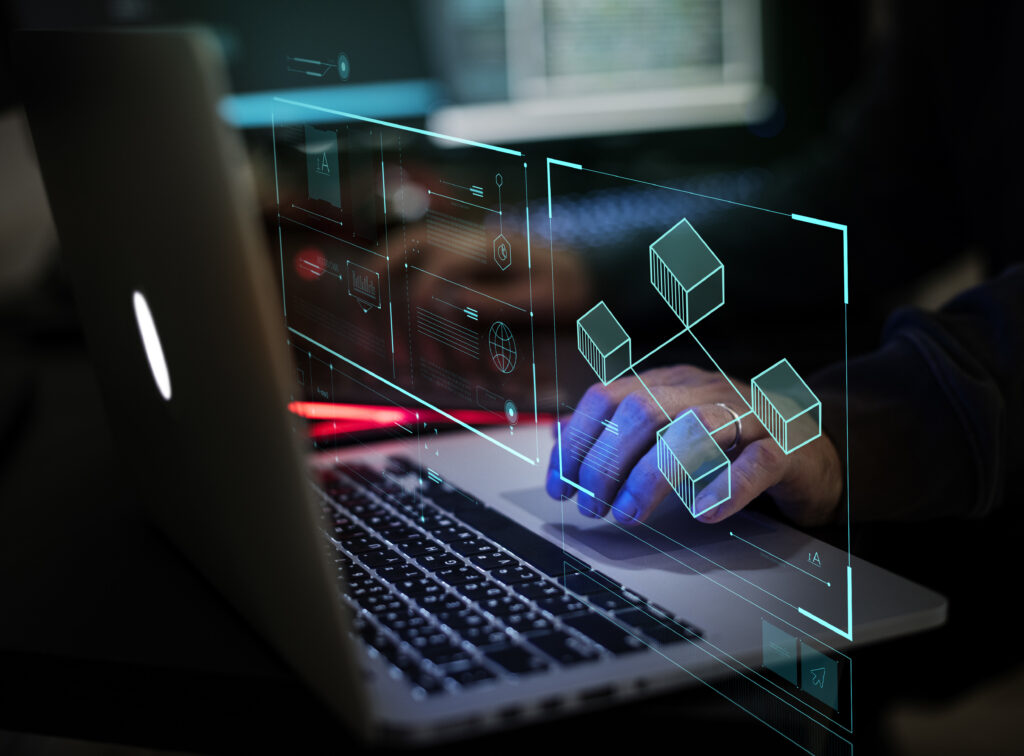
2. Phishing Detection and Response
In the realm of phishing detection and response, Generative AI systems offer unparalleled precision. These systems learn from vast datasets of legitimate and malicious communications to distinguish between them effectively. This learning enables the AI to identify subtle signs of phishing attempts that might escape human analysts. Additionally, once a threat is detected, these systems can automatically initiate responses such as isolating affected email accounts and alerting users, mitigating phishing attacks swiftly and efficiently.
Moreover, Generative AI can simulate phishing attacks to train both the AI systems and the employees, enhancing their ability to recognize and respond to phishing attempts. This ongoing training process continually improves the detection algorithms and equips employees with up-to-date knowledge, significantly reducing the likelihood of successful phishing attacks.
3. Automated Security Protocol Testing
Generative AI excels in the automated testing of security protocols by identifying vulnerabilities in networks and systems before attackers can exploit them. Through comprehensive simulations and stress tests, Generative AI systems evaluate the robustness of security measures and pinpoint weaknesses. This proactive testing is essential for maintaining the integrity of an organization’s cybersecurity infrastructure.
The iterative process of testing and retesting, powered by AI, ensures that security protocols evolve to counter new and emerging threats. By automating these tests, organizations can regularly update their defense mechanisms without substantial manual oversight, maintaining a high-security standard with greater efficiency.
4. Incident Response
AI-driven tools revolutionize incident response by providing automated, rapid, and data-driven decision-making during security breaches. These tools analyze the scale and scope of an incident in real-time and recommend or initiate actions to mitigate damage, such as isolating infected network segments or deploying security patches. The speed and accuracy of AI-driven responses often exceed what is possible through human intervention alone, reducing downtime and the potential for significant data loss.
Further, Generative AI can simulate various breach scenarios to train response teams, ensuring they are prepared for multiple incidents. This preparation is critical for developing effective recovery plans and can dramatically shorten response times when actual incidents occur.
5. Behavioral Biometrics
Generative AI enhances security by analyzing behavioral patterns to detect anomalies that may indicate a breach, such as unusual login times or locations. Behavioral biometrics is crucial in identifying compromised user accounts and insider threats. By continuously learning and adapting to new behaviors, AI systems can provide a dynamic security measure that complements traditional authentication methods.
Such systems are particularly effective in environments where sensitive information is handled, as they can detect subtle changes in behavior that might signify malicious intent or a compromised account, thus enabling preemptive action to secure the systems.
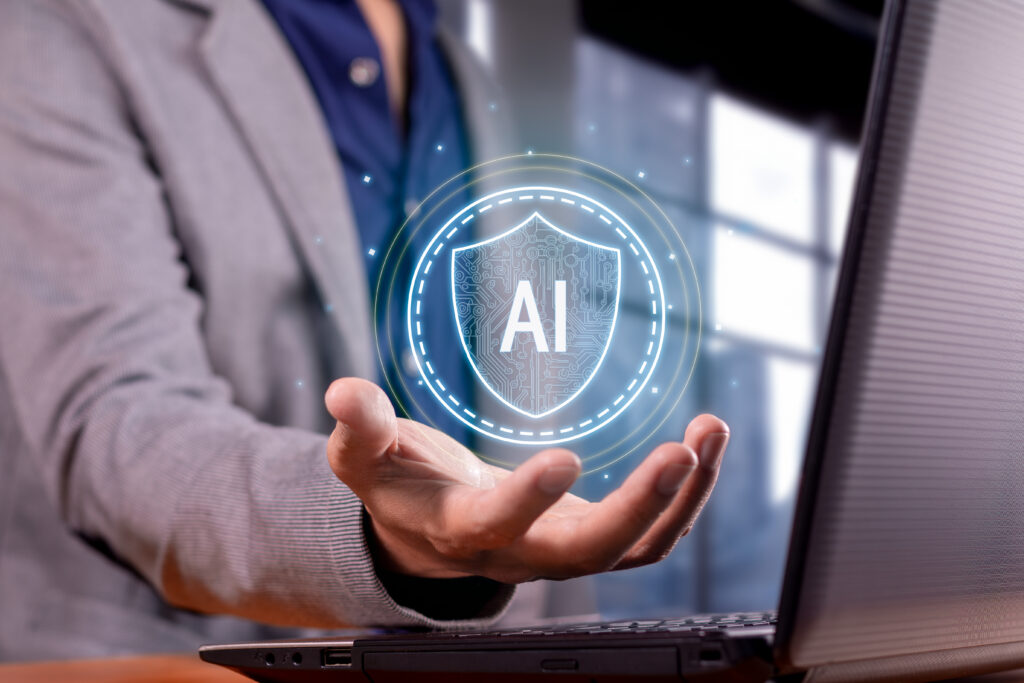
6. Security Training and Awareness Programs
Generative AI is also critical in developing customized training and awareness programs. By analyzing past security incidents and current trends, AI systems can design training modules that address specific vulnerabilities within an organization. These programs are tailored to the organization’s unique needs, ensuring that all employees are well-equipped to recognize and respond to cybersecurity threats.
The adaptability of AI-driven training programs means they can continually evolve based on new data, ensuring that training remains relevant as new threats and technologies emerge. This ongoing education is vital for maintaining an informed and vigilant workforce capable of defending against sophisticated cyber-attacks.
Challenges and Considerations in Generative AI for Cybersecurity
Integrating Generative AI into cybersecurity, while offering vast benefits, also brings a host of ethical considerations and potential risks. As these technologies become more embedded in security operations, the implications for data privacy, misuse, and AI-generated attacks need careful consideration. This section explores these challenges in-depth, providing a framework for addressing ethical dilemmas in deploying Generative AI tools in cybersecurity.
Data Privacy and Integrity
Data privacy is one of the foremost ethical concerns with the use of Generative AI in cybersecurity. AI systems require access to vast amounts of data to learn and make informed decisions. This data often includes sensitive information, which, if mishandled, could lead to significant privacy breaches. Ensuring that Generative AI systems adhere to strict data protection standards and regulations is crucial. Additionally, there is the issue of data integrity—ensuring that the data used to train AI models is accurate, unbiased, and not manipulated by malicious actors. The integrity of training data is essential, as compromised data can lead to flawed decisions, further complicating the ethical landscape.
Risk of AI-Generated Attacks
Another significant risk associated with Generative AI is the potential for these technologies to create sophisticated cyber-attacks. AI models that can generate phishing emails, mimic human behavior, or create malware are double-edged swords. In the wrong hands, these capabilities could enhance the effectiveness of cyber-attacks, making them more challenging to detect and counter. This potential makes it imperative to establish robust governance frameworks that restrict the misuse of AI technologies and ensure they are used solely for defensive purposes.
Ethical Deployment and Use
The deployment of Generative AI tools must be guided by ethical principles that prioritize human welfare and the protection of digital assets. This involves the ethical development and testing of these tools and their implementation in a manner that respects user privacy and data security. Transparency in how AI systems make decisions, particularly in incident response and threat detection, is vital to maintaining trust and accountability.
Reflections on Ethical Deployment
In analyzing the ethical deployment of AI tools in cybersecurity, it is clear that maintaining a balance between innovation and ethical responsibility is paramount. This balance ensures that while cybersecurity capabilities are enhanced, they do not inadvertently cause harm or create new cyber threats. Furthermore, there must be an ongoing dialogue among cybersecurity professionals, lawmakers, and AI developers to continuously evaluate the ethical implications of new technologies. This collaborative approach ensures that ethical considerations keep pace with technological advancements.
Conclusion: Embracing the Future of Generative AI in Cybersecurity
The transformative potential of Generative AI in cybersecurity is undeniable. This advanced technology offers many benefits, from enhancing threat detection and automating responses to fostering predictive capabilities that anticipate future attacks. As we have explored throughout this article, Generative AI can revolutionize how cybersecurity professionals approach and manage cyber threats, providing tools that are not only reactive but also proactive and predictive. The ability to generate and analyze vast amounts of data can significantly accelerate the identification of potential threats and vulnerabilities, ensuring that defenses are robust and adaptable.
However, the rapid advancement and integration of Generative AI into cybersecurity frameworks also necessitate heightened vigilance and adaptation. The dynamic nature of AI technology means that cybersecurity strategies must continuously evolve to keep pace with technological advancements and potential threats. The ethical considerations and risks associated with AI, such as data privacy concerns and the possibility of AI-generated attacks, require ongoing attention and careful management. Ensuring these technologies are used responsibly and ethically must be a priority for all stakeholders.
Furthermore, the cybersecurity community must remain vigilant against complacency. As AI technologies become more embedded in security operations, there is a risk that reliance on automated systems could lead to gaps in human oversight. Continuous education and training for cybersecurity professionals are essential to maintain a critical balance between human expertise and AI capabilities. This balance is crucial for effective threat management and the ethical deployment of AI tools.
In conclusion, while Generative AI holds the remarkable potential to fortify cybersecurity defenses, its deployment must be accompanied by continuous adaptation and rigorous oversight. By embracing these technologies with an informed and cautious approach, the cybersecurity community can ensure that AI remains a boon, not a bane, to their efforts. This balance of innovation, ethics, and vigilance will be key to navigating the future challenges of cybersecurity, making digital environments safer for everyone.
References:
- Das, R. (2024) Generative AI: Phishing And Cybersecurity Metrics (Cyber Shorts). 1st ed. Boca Raton: CRC Press.
- Harvard Business Review and Kaye, R. (2024) Generative AI: The Insights You Need from Harvard Business Review (HBR Insights Series). Boston: Harvard Business Review Press.
- Yadav, D. (2023) Generative AI: A Double-Edged Sword for Data Security.